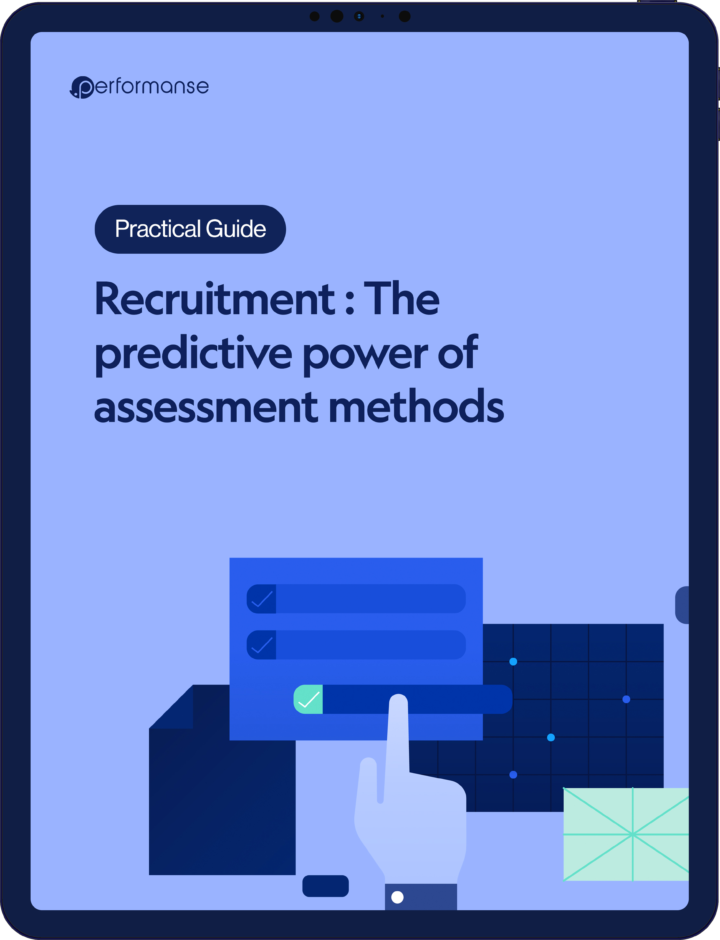
We would all like to be able to predict the success of our candidates or employees (in a position, within a company) as well as meteorologists predict the weather.
While this is not yet fully achievable, the gap is narrowing. This is notably thanks to the evolution of evaluation methods, now enriched by technological advancements.
Our guide, the result of in-depth studies, is dedicated to evaluation methods that have been assessed and scientifically validated for their predictive capacity. You will discover a detailed scoring of each method, allowing you to understand their level of reliability and effectiveness in the current professional context.
More than just a compilation, this guide is a reflection on the ongoing innovations in the world of human resources, particularly with the emergence of generative AI and advanced technologies. We explore how these developments could influence and improve prediction scores, paving the way for even more precise and personalized practices in talent management.
This guide is an essential resource for anyone interested in the future of recruitment and performance management. It lays the groundwork for understanding where we are today and how we can prepare for the transformations that the future holds in the field of human potential prediction.
Happy reading!
Newsletter
Thank you for subscribing to our newsletter!
You’ll now receive our articles, be informed of our events and benefit from our white papers.
Ready to discover your next talents?
Complete the form to get a demo with one of our experts
Footer form
Thank you!
Your info was submitted successfully.